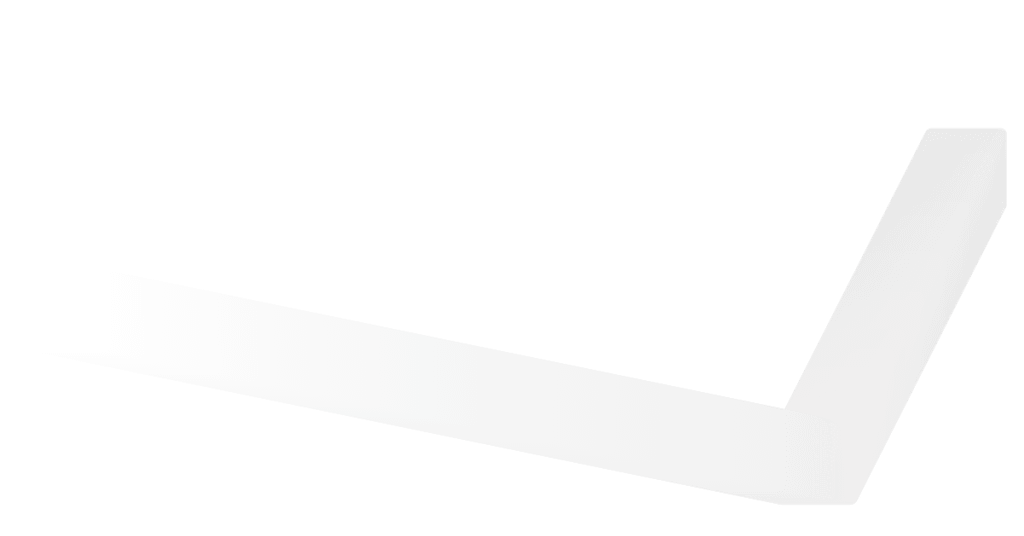
What is AI Model Pricing?
AI model pricing refers to the pricing strategy used for artificial intelligence (AI) solutions, where the price is determined based on factors such as the complexity of the model, usage, the value provided, and the underlying computational resources required to operate the model. This pricing strategy is used by businesses offering AI-powered products and services, including SaaS providers, machine learning platforms, and AI consultants. AI model pricing is an important consideration in industries like software, healthcare, finance, and marketing, where AI solutions can range from simple tools to highly complex models.
In the context of SaaS and AI-powered software, AI model pricing typically varies depending on the usage of the model, the amount of data processed, the complexity of the algorithms, and the computing power required. For example, a company offering a machine learning model for predictive analytics might charge based on the number of API calls made to access the model, or the volume of data processed through the model. Alternatively, they might offer tiered pricing, where customers pay for access to different levels of model complexity, with more advanced models being priced at a premium.
One of the core principles behind AI model pricing is aligning the price with the value delivered by the AI solution. In many cases, AI models offer significant cost savings or improvements in efficiency, so businesses want to ensure their pricing reflects the value customers receive. For instance, a recommendation engine model that helps an e-commerce company increase sales might be priced based on the incremental revenue it generates for the customer. Similarly, a fraud detection model used in banking or finance might be priced based on the cost savings it offers by reducing fraudulent transactions.
AI model pricing can also be based on the level of customization required for a specific customer. Businesses offering AI models may provide pricing tiers that include off-the-shelf models, which are pre-trained and ready to use, and custom models, which are built specifically for the customer's needs. Custom AI models often command higher prices due to the time and resources involved in their development, as well as the need for ongoing support and updates.
From a sales perspective, AI model pricing requires an understanding of both the customer’s needs and the potential ROI the AI solution offers. Sales teams must be able to effectively communicate the benefits of the AI model, its scalability, and its ability to generate value. The sales process may involve discussing different pricing options, such as pay-per-use models, subscription pricing, or pricing based on the number of users or data volume. For example, a software company offering an AI-powered analytics platform might offer pricing based on the number of monthly users or the amount of data ingested into the platform, while also offering premium features or model customization at a higher price point.
For finance teams, AI model pricing provides an opportunity to optimize revenue while ensuring that the pricing structure remains sustainable and competitive. Finance teams need to analyze the cost structure of delivering AI solutions, including the costs of training the models, maintaining them, and the cloud infrastructure required to deploy them. These factors help determine the most profitable pricing models, whether it’s a usage-based model, subscription-based pricing, or a hybrid approach. Additionally, finance teams must account for the potential for price differentiation based on the complexity and scale of the AI model.
One of the challenges with AI model pricing is ensuring fairness and transparency. Customers may be hesitant to adopt AI solutions if they feel the pricing is unclear or based on opaque factors. Businesses offering AI models need to provide clear explanations of how pricing is determined and ensure that customers understand what they are paying for. Providing a clear breakdown of costs, such as usage fees, processing power costs, and premium features, can help build trust and foster long-term relationships with customers.
Another consideration in AI model pricing is the ongoing support and updates that customers may require. AI models, particularly those used for mission-critical applications, need to be continuously maintained and improved. Offering support packages or subscription services for updates and model retraining may be part of the pricing strategy, adding recurring revenue streams for the business.
Ultimately, AI model pricing requires a careful balance between offering competitive pricing that reflects the value of the AI solution while ensuring profitability for the business. By considering factors like model complexity, data usage, customer needs, and value delivery, businesses can implement pricing strategies that optimize both revenue and customer satisfaction. As the AI industry continues to evolve, businesses will need to stay agile and adjust their pricing models to meet the demands of the market and their customers.
Looking to solve monetization?
Learn how we help fast-growing businesses save resources, prevent revenue leakage, and drive more revenue through effective pricing and billing.
Absorption Pricing
Accounts Receivable
ACH
Advance Billing
AI Agent Pricing
AI Model Pricing
AI Token Pricing
AISP
ARR
ASC 606
Automated Investment Services
Automated Invoicing
Basing Point Pricing
Basket-based Pricing
Billing Cycle
Billing Engine
Captive Product
Channel Incentives
Channel Pricing
Choke Price
Churn
Clearing and Settlement
Commercial Pricing
Competitive Pricing
Consolidated Billing
Consumption Based Pricing
Contribution Margin-Based Pricing
Conversation Based Pricing
Cost Plus Pricing
Cost-Based Pricing
CPQ
Customer Based Pricing
Customer Profitability
Deal Management
Deal Pricing Guidance
Deal Pricing Optimization
Decoy Pricing
Deferrred Revenue
Digital Banking
Discount Management
Dual Pricing
Dunning
Dynamic Pricing
Dynamic Pricing Optimization
E-invoicing
E-Money
EBIDTA
Embedded Finance
Enterprise Resource Planning (ERP)
Entitlements
ERP
Feature-Based Pricing
Finance AI
Fintech
Fintech Ecosystem
Flat Rate Pricing
Freemium Model
Frictionless Sales
Generative AI Pricing
Grandfathering
Guided Sales
Hedonic Pricing
High-Low Pricing
Hybrid Pricing Models
Idempotency
IFRS 15
Insurtech
Intelligent Pricing
Invoice
Invoice Compliance
KYC
Lending-as-a-Service (LaaS)
Lifecycle Pricing
Loss Leader Pricing
Margin Leakage
Margin Management
Margin Pricing
Marginal Cost Pricing
Market Based Pricing
Metering
Micropayments
Minimum Commit
Minimum Invoice
MRR
Multi-currency Billing
Multi-entity Billing
Neobank
Net Dollar Retention
Odd-Even Pricing
Omnichannel Pricing
Open Banking
Outcome Based Pricing
Overage Charges
Pay What You Want Pricing
Payment Gateway
Payment Processing
Peer-to-peer Lending
Penetration Pricing
PISP
Predictive Pricing
Price Benchmarking
Price Configuration
Price Elasticity
Price Estimation
Pricing Analytics
Pricing Bundles
Pricing Efficiency
Pricing Engine
Pricing Software
Product Pricing App
Proration
PSD2
PSP
Quotation System
Quote Request
Quote-to-Cash
Quoting
Ramp Up Periods
Real-Time Billing
Recurring Payments
Region Based Pricing
RegTech
Revenue Analytics
Revenue Backlog
Revenue Forecasting
Revenue Leakage
Revenue Optimization
Revenue Recognition
SaaS Billing
Sales Enablement
Sales Optimization
Sales Prediction Analysis
SCA
Seat-based Pricing
Self Billing
Smart Metering
Stairstep Pricing
Sticky Stairstep Pricing
Subscription Management
Supply Chain Billing
Tiered Pricing
Tiered Usage-based Pricing
Time Based Pricing
Top Tiered Pricing
Total Contract Value
Transaction Monitoring
Usage Metering
Usage-based Pricing
Value Based Pricing
Volume Commitments
Volume Discounts
WealthTech
White-label Banking
Yield Optimization
Why Solvimon
Helping businesses reach the next level
The Solvimon platform is extremely flexible allowing us to bill the most tailored enterprise deals automatically.
Ciaran O'Kane
Head of Finance
Solvimon is not only building the most flexible billing platform in the space but also a truly global platform.
Juan Pablo Ortega
CEO
I was skeptical if there was any solution out there that could relieve the team from an eternity of manual billing. Solvimon impressed me with their flexibility and user-friendliness.
János Mátyásfalvi
CFO
Working with Solvimon is a different experience than working with other vendors. Not only because of the product they offer, but also because of their very senior team that knows what they are talking about.
Steven Burgemeister
Product Lead, Billing